Navigating AI Accuracy in Digital Evidence Management
by Malaika Batool, Last updated: November 4, 2024, Code:
AI offers tantalizing promises, especially in fields that require intensive handling and organization of digital evidence, such as law enforcement, legal services, and corporate compliance. For professionals working with sensitive data, the potential of AI to automate redaction, organize files, and manage complex video content is irresistible. However, despite AI’s capabilities, the expectations placed on it are often misaligned with reality.
When managing evidence and sensitive video content, accuracy is paramount, and while AI is a powerful tool, its limitations must be acknowledged and carefully managed. A survey conducted by McKinsey reported that 44% of respondents said their organization had experienced at least one negative consequence due to AI inaccuracy. Setting realistic expectations is crucial for leveraging AI effectively in these high-stakes fields, where errors could lead to costly consequences, legal challenges, or data privacy breaches.
In this guide, we’ll explore the realities of AI’s accuracy in digital evidence management video redaction, and content organization, providing insights into its potential and limitations. By understanding the complexities of AI in these areas, professionals can adopt best practices that maximize AI’s strengths while safeguarding against its weaknesses.
Understanding the Stakes: Why Precision Is Non-Negotiable
In digital evidence management, accuracy isn’t a luxury—it’s a requirement. For legal, law enforcement, and corporate compliance roles, accuracy underpins trust, fairness, and security. A seemingly minor error in redaction or evidence categorization can have severe implications.
Imagine the scenario: a prosecutor preparing for trial accidentally shares sensitive information in an unredacted video. Or consider a compliance officer who relies on AI to tag evidence only to discover that it misidentified critical elements. These are not merely procedural inconveniences; they have profound impacts on legal outcomes, regulatory compliance, and organizational integrity.
Every field that uses AI for digital evidence management has specific requirements:
- Law Enforcement: Inaccurate redaction or tagging could compromise investigations, damage public trust, or even result in wrongful convictions.
- Legal Services: Missed redactions or misclassified evidence can bias a trial or lead to appeals, increasing both the cost and duration of legal proceedings.
- Corporate Compliance: In industries with stringent regulations, inaccurate handling of evidence can lead to non-compliance, penalties, and significant reputational harm. The ramifications of error in these areas make it clear that accuracy cannot be assumed. While AI has advanced, it remains imperfect, and professionals must use it strategically to avoid pitfalls.
The Realities of AI Accuracy in Digital Evidence Management
The Foundations of AI Accuracy
AI’s accuracy in digital evidence management is only as strong as the data it’s trained on. High-quality data allows AI to analyze patterns, categorize content, and perform redactions, but its effectiveness diminishes when dealing with substandard data. For instance, an AI system trained only on high-resolution footage may falter when analyzing low-light or low-resolution video typical of certain surveillance feeds. Additionally, data that lacks diversity can introduce biases, skewing AI’s interpretation of sensitive evidence.
Training models specifically for digital evidence management can enhance performance but requires significant resources and expertise. Custom training helps improve AI’s ability to recognize relevant patterns and accurately tag metadata, yet even with such training, human oversight is essential to catch subtle errors. While AI can handle repetitive tasks like sorting and tagging, it lacks the contextual understanding needed for accurate decision-making in nuanced scenarios, such as distinguishing similar objects or identifying specific legal thresholds.
Video Redaction
AI-driven video redaction holds enormous promise, especially in fast-paced environments where rapid redaction can save hours or even days. However, speed doesn’t always mean precision. Redaction accuracy can be compromised when videos contain complex scenes, low lighting, or rapid movement. For example, AI may mistakenly miss redactions in footage where multiple faces appear in motion, or it might blur non-sensitive areas, obscuring crucial information.
Over-redaction and under-redaction are two common challenges in video redaction. Over-redaction leads to unnecessary blurring, which can compromise the integrity of the evidence by concealing non-sensitive details. Under-redaction, on the other hand, can expose sensitive information, creating compliance risks. While AI can handle basic redaction needs quickly, hybrid workflows that combine AI’s efficiency with human review are essential for high-stakes content.
Video Content Management
Video content management, particularly for legal and compliance uses, benefits from AI’s ability to automate categorization and tagging, making it easier to search and retrieve relevant content. However, the limitations of AI become apparent in areas requiring deep semantic understanding. For example, AI might miscategorized footage based on surface-level features or tag scenes incorrectly if it cannot discern context. This can result in incorrect or incomplete data that makes it harder to locate crucial information during investigations or trials.
AI can reliably handle simple searches, but it struggles with complex content analysis that requires contextual insight. For instance, when a compliance officer searches for a particular event in video footage, AI may fail to identify it accurately if the scene’s elements are subtle or visually complex. Manual verification of tagged content is often necessary to ensure accuracy, particularly in legal scenarios where evidence misclassification can have legal ramifications.
Why Overestimating AI Capabilities Can Be Dangerous
Over-Reliance on AI in High-Stakes Decisions
Many organizations have mistakenly placed total confidence in AI for managing and reviewing digital evidence, assuming it to be foolproof. However, AI is not infallible, and overestimating its abilities can lead to inadequate oversight and critical errors. In legal or compliance settings, where decisions based on AI can impact people’s lives, rights, or organizational reputation, the risks of AI-driven mistakes are amplified.
Human review is a crucial component of AI-driven workflows. In a court case, for example, AI could misidentify a piece of evidence or inadvertently redact critical information. Such errors are especially concerning when organizations assume AI’s output to be flawless, leading to false confidence and minimal oversight.
Data Privacy Risks and Compliance Challenges
Organizations using AI for evidence and video management are bound by data privacy regulations like the GDPR, CCPA, and HIPAA. AI-driven tools must therefore ensure that sensitive information is consistently redacted or anonymized. However, AI’s limitations can sometimes result in redaction errors, leading to unintentional privacy violations. For instance, if an AI tool misses the faces of bystanders in a surveillance video, this could breach privacy requirements, resulting in potential fines and reputational damage.
In this context, relying solely on AI for compliance-sensitive tasks is risky. Organizations must have policies in place for human review and quality checks to verify that redactions meet regulatory standards. Failing to do so could mean financial and legal consequences if sensitive data is exposed due to AI’s oversight. Choosing software that allows for manual redaction or review process along with AI-capabilities comes handy in such situations.
Ethical and Legal Implications of AI in Evidence Management
AI’s reliance on algorithms rather than contextual judgment can introduce bias or inaccuracies, raising ethical concerns. Bias in evidence management can distort investigations or impact case outcomes. For example, AI algorithms trained on unbalanced datasets may produce biased evidence categorizations or redactions, affecting the fairness of legal processes.
To prevent such issues, organizations must implement strict guidelines for AI use and ensure ongoing evaluations to detect and correct potential biases. In high-stakes fields like law and compliance, these ethical and legal implications cannot be ignored, making transparency and human oversight essential in managing AI outputs.
Strategies for Effective AI Use in Digital Evidence Management
Hybrid Workflows for Redaction: Leveraging AI with Human Oversight
One effective approach to AI-driven video redaction is implementing hybrid workflows. While AI excels at bulk redactions, human reviewers are vital for confirming accuracy and refining details in high-stakes content. For example, AI can perform an initial pass on footage to redact faces, license plates, and confidential information, but human review ensures that sensitive details aren’t missed. This combination allows professionals to save time without sacrificing precision, balancing AI’s efficiency with human judgment.
Custom Training to Improve Model Accuracy
Training AI models on datasets specific to digital evidence management helps increase accuracy. By customizing models with data representative of actual evidence, AI can learn to better recognize patterns and features relevant to your needs. For example, law enforcement agencies might train AI on crime scene footage to improve its ability to identify key elements like weapons or persons of interest. Although training requires initial time and resource investments, it can significantly enhance AI’s accuracy in the long term.
Regular Monitoring and Quality Control for Consistent Performance
Regularly monitoring AI’s performance is essential for maintaining accuracy and accountability. Establishing quality control benchmarks—such as accuracy thresholds in redaction or categorization—helps teams catch and correct issues early on. Quality control measures also allow professionals to evaluate whether AI-generated metadata and tags align with expectations. Regular testing on varied content ensures that AI continues to perform as intended, making it a more reliable tool in the long run.
Safeguarding Data Privacy with Manual Reviews
To avoid data privacy risks, organizations should not rely solely on AI for sensitive redactions. Manual reviews help identify missed redactions, verifying that all private information is appropriately obscured. Regular audits of AI tools further strengthen compliance by confirming that all redactions meet legal standards. Human oversight at key stages of the redaction process ensures compliance with privacy regulations and reduces exposure to legal risks.
Defining Clear Guidelines for AI Use
Setting clear guidelines for AI use in evidence management helps professionals understand when human intervention is needed. Clear policies ensure that teams approach AI with realistic expectations, making it easier to integrate AI efficiently while mitigating risks. For example, guidelines may specify that all legal-sensitive footage undergoes manual review post-redaction or outline procedures for handling AI misclassifications.
Conclusion: Embracing AI’s Benefits with Realistic Expectations
AI is a powerful tool that can significantly improve efficiency in digital evidence management, but it is not a silver bullet. Human expertise and oversight remain crucial, especially for tasks that require judgment and contextual understanding. By setting realistic expectations, organizations can leverage AI effectively while avoiding the pitfalls of over-reliance. In fields as sensitive as law and compliance, AI works best as a supportive tool rather than a replacement for human expertise.
In the coming years, AI models will likely continue to improve in their ability to handle complex datasets and understand nuanced content. However, the hybrid approach—where AI works alongside human reviewers—will likely remain the most effective strategy in high-stakes environments. By balancing AI’s efficiency with human oversight, organizations can maximize accuracy and reliability, benefiting from AI’s capabilities without compromising integrity.
People Also Ask
How accurate is AI in handling digital evidence?
AI can accurately perform tasks such as tagging, sorting, and metadata generation, but complex or sensitive evidence typically requires human oversight to ensure complete accuracy.
Can AI fully automate video redaction in legal cases?
No. While AI can expedite redaction tasks, human review is essential to catch details AI might miss, particularly in complex or fast-moving footage.
How can AI accuracy in evidence management be improved?
Organizations can enhance AI’s performance through custom training, regular monitoring, and setting clear quality control benchmarks.
Are there any legal standards for using AI in evidence management?
Standards vary, but best practices include regular audits, compliance checks, and clearly defined guidelines for AI use.
What ethical considerations come with using AI in evidence management?
Potential biases and inaccuracies can impact legal fairness and compliance, so transparency and human oversight are essential.
Jump to
You May Also Like
These Related Stories
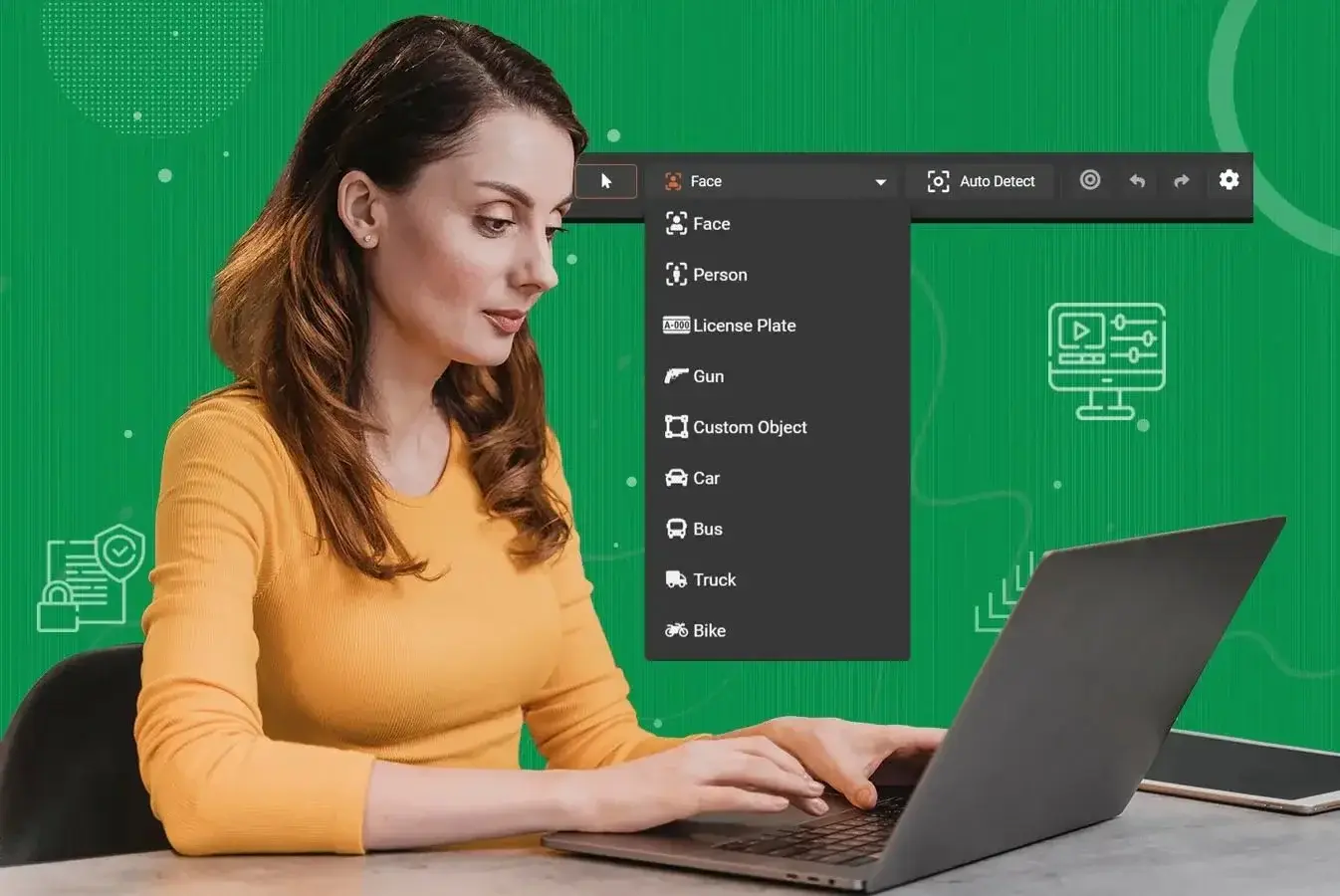
How to Redact a Video Efficiently with Video Redaction Software
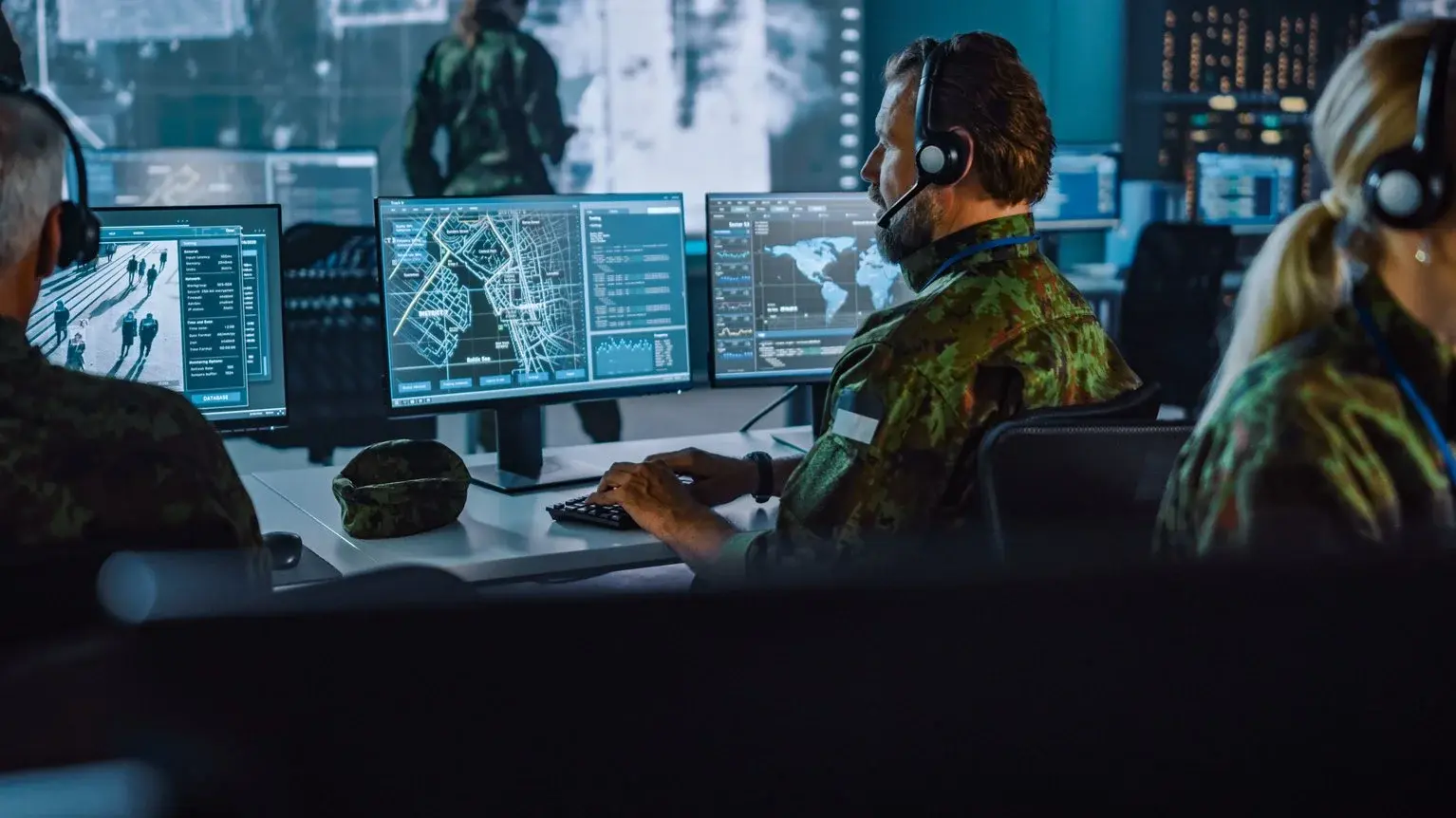
15 Ways Law Enforcement Digital Evidence Management is Transforming Police Data Handling
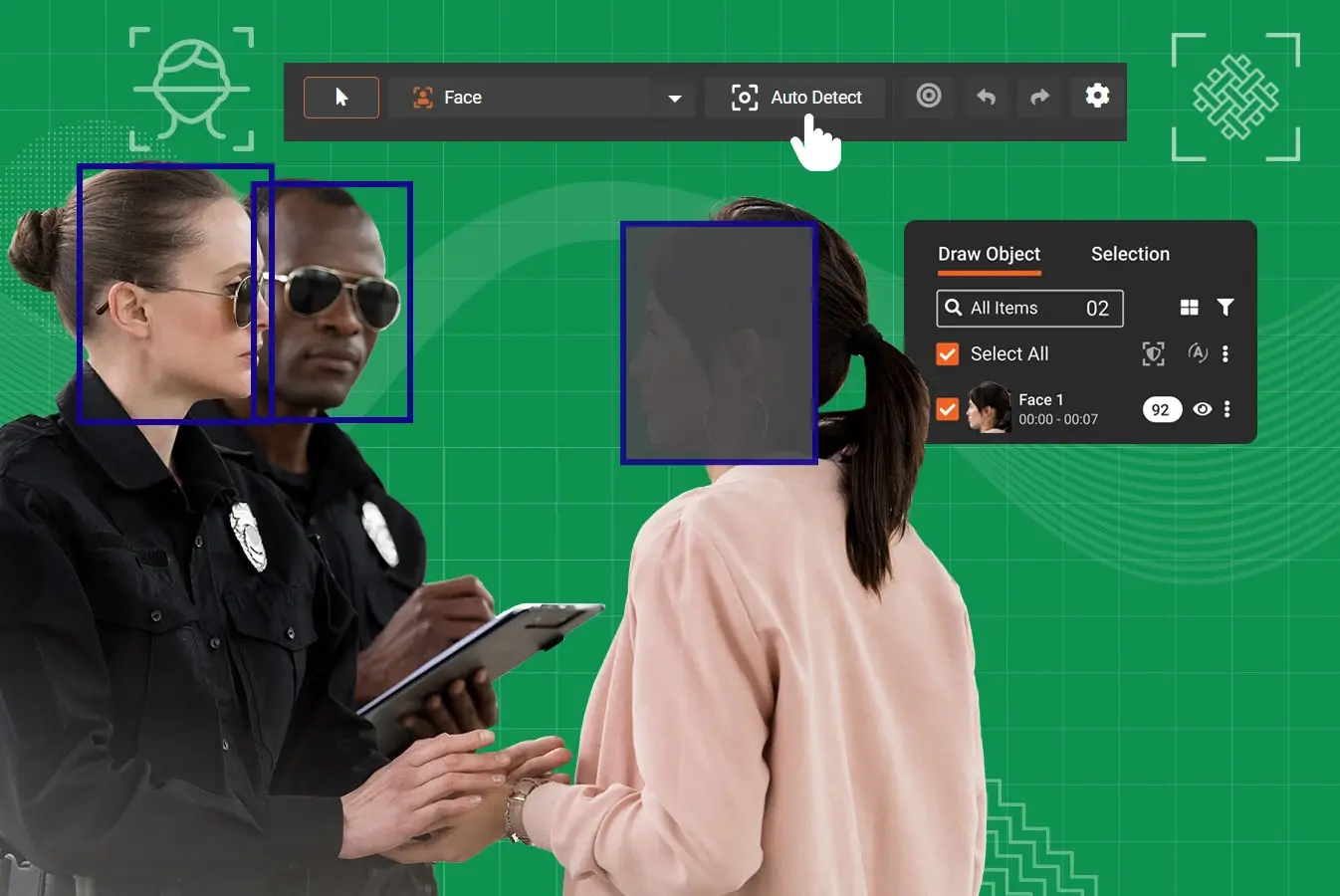
No Comments Yet
Let us know what you think