AI Model Matchmaker: Choosing the Right Model for Your Business Needs
by Rafey Iqbal Rahman, Last updated: November 13, 2024, Code:
You’ve been tasked with leveraging AI to drive your business forward. But here’s the problem: the AI landscape is more crowded than ever, and choosing the right model feels like picking a needle out of a haystack. You’re not just gambling with tech. You're gambling with your company’s future. That’s a lot of pressure to get it right, isn’t it?
You know you need AI, but the array of models, jargon, and use cases leaves you overwhelmed. Machine learning, neural networks, deep learning, they all sound great on paper. But how do you match the right AI model to your actual business needs? And how do you avoid investing in a solution that’s either too complicated, too simple, or completely misaligned with your goals?
Let’s cut through the noise. This guide is your AI matchmaker, helping you choose the model that meets your business objectives without the headache.
The AI Overload Dilemma
The AI revolution promises groundbreaking results: predictive analytics, automation, and customer insights. But here’s the thing no one talks about. You can’t just plug in any AI model and expect magic to happen. It’s like buying a Ferrari for city traffic. Sure, it’s impressive, but it’s overkill for the task at hand.
The real issue is that AI doesn’t come in one-size-fits-all. With various models, each with its own set of strengths, weaknesses, and ideal use cases, choosing the wrong one can mean wasted resources, delayed implementation, and disappointing results. Worse, it could set your AI strategy back months, if not years.
The flood of AI solutions has also created a paralysis by analysis. CIOs, CTOs, and data science leads face so many choices that many defer decisions, hoping that clearer answers will emerge. But time is ticking. Competitors are already deploying AI models, and every delay is an opportunity lost.
The Missteps and Pitfalls of AI Model Selection
Let's assume this. You invest in a powerful neural network for predictive analytics, but your data isn't sufficient to feed the model. The result? A model that’s essentially useless, unable to deliver accurate predictions. Or maybe you choose a more basic decision tree algorithm because it’s easy to deploy, only to find it lacks the sophistication to solve your business's nuanced problems.
In both cases, you’ve spent time, money, and resources, but the outcome is the same: failure to deliver.
The pain of AI missteps is real. And it’s not just the immediate financial cost, it’s the trust of your stakeholders. The CIO who trusted you to lead the digital transformation, the CTO who bet on AI to enhance the tech stack, and the product manager counting on AI to bring innovation to the product pipeline. They’re all watching, and they expect results.
Moreover, even when you think you’ve made the right choice, issues like scalability, integration, and maintenance often crop up unexpectedly. The AI model that seemed so promising in the demo doesn’t mesh well with your legacy systems. It doesn’t scale as your data grows. Suddenly, what started as a leap into the future has become a series of stumbling blocks.
The AI Matchmaking Process
So, how do you avoid these AI selection pitfalls? You need a structured approach—a matchmaking process that aligns your business goals with the capabilities of various AI models. Here’s how to do it.
Start with Your Business Problem
Before thinking about AI models, define the business problem you’re trying to solve. Are you trying to predict customer behavior? Automate manual tasks? Detect fraud?
Your business problem will directly influence the type of AI model you should consider. For example, if your goal is to predict outcomes based on historical data, you might need a model specializing in predictive analytics, like a regression model or decision tree. If your goal is to analyze large datasets for insights, deep learning might be a better fit.
It’s tempting to start with the shiny new tech. After all, who doesn’t want to implement the latest neural network? However, doing so without first understanding the business context is a recipe for failure.
Understand the AI Model Types and Their Best Uses
AI models generally fall into a few key categories. Here’s a breakdown of the most common types and when to use them:
- Decision Trees: Simple and interpretable, decision trees work well for tasks like classification and regression. They’re great when transparency and explainability are important, such as in highly regulated industries like finance and healthcare.
- Linear Regression: Perfect for predictive tasks where you need to model relationships between variables. Think sales forecasting or customer demand prediction.
- Support Vector Machines (SVMs): Ideal for classification tasks with smaller datasets. SVMs are often used in text categorization and image recognition.
- Neural Networks: These excel at handling complex, high-dimensional data. A neural network might be the best choice if you’re working on image recognition, natural language processing, or even some predictive analytics tasks. However, neural networks require significant data and computational power.
- Deep Learning: An extension of neural networks, deep learning models are best suited for complex tasks like image and speech recognition. However, they are resource-intensive and may be overkill for simpler tasks.
- Random Forests: A versatile option, random forests combine multiple decision trees to increase accuracy. They’re great for tasks like risk assessment, fraud detection, or even personalized recommendations.
Understanding these model types and their applications can help you narrow down your options based on the specific problems you're trying to solve.
Assess Your Data Readiness
No AI model can work without the right data, no matter how sophisticated. Ask yourself:
- Do you have enough data to train the model effectively?
- Is your data clean, structured, and accessible?
- Are there gaps in your data that could limit the model’s performance?
Data readiness is critical. Even the most advanced AI model will struggle to deliver accurate results if your data isn't up to par.
Match the Model to Your Operational Capabilities
Just as you wouldn’t buy a sports car if you live in an area with poor roads, don’t choose an AI model that exceeds your operational capabilities. Consider factors like:
- Scalability: Can the model scale as your data grows, or will it require constant updates and maintenance?
- Computational Power: Does your existing tech infrastructure support the model’s computational needs, or will you need to invest in upgrades?
- Integration: Will the model integrate smoothly with your existing systems, or will you need to rework your entire tech stack?
Choosing an AI model isn’t just about what it can do but what your business can handle.
Consider Futureproofing and Flexibility
The pace of AI evolution is rapid. While it’s essential to solve today’s business problems, you also need to consider how adaptable the model will be in the future. Does it allow for:
- Easy updates as new data becomes available?
- Modularity, enabling you to add features or adapt to new use cases?
- Long-term sustainability, ensuring that the model can evolve with your business?
You don’t want to be stuck with an AI model that works today but becomes obsolete tomorrow.
The Right AI Model is the One that Solves Your Business Problem
Selecting the right AI model can feel like navigating a maze, but it doesn’t have to be. By focusing on your business needs, understanding the strengths and weaknesses of different AI models, assessing your data readiness, and considering your operational capacity, you can avoid common pitfalls and choose a model that delivers real business value.
Remember, the key to AI success isn’t just choosing the most powerful model—it’s about choosing the model best suited to your unique business challenges.
People Also Ask
What are the most important factors when selecting an AI model for my business?
Focus on the specific business problem you're solving, the type of data you have, and your operational capabilities.
Can I use AI without large datasets?
Yes, models like decision trees and support vector machines can work well with smaller datasets.
How do I know if my data is ready for AI?
Your data should be clean, well-structured, relevant to the problem you're solving, and sufficient to train the model.
What’s the difference between machine learning and deep learning?
Machine learning focuses on algorithms that can learn from data. Deep learning, a subset of machine learning, uses neural networks with many layers to handle complex tasks like image or speech recognition.
How do I future-proof my AI investment?
Choose flexible models that can be updated with new data and integrated into a modular tech stack for scalability.
Is AI expensive to implement?
AI implementation costs can vary, but starting small with specific models and scaling as needed can effectively manage costs.
What’s the difference between supervised and unsupervised learning models?
Supervised learning requires labeled data and is used for tasks like classification. Unsupervised learning doesn’t need labeled data and is used for clustering and discovering patterns.
How long does it take to see results from AI?
Results vary depending on the model's complexity and the data's quality. Some businesses see results in weeks, while others may take months.Jump to
You May Also Like
These Related Stories
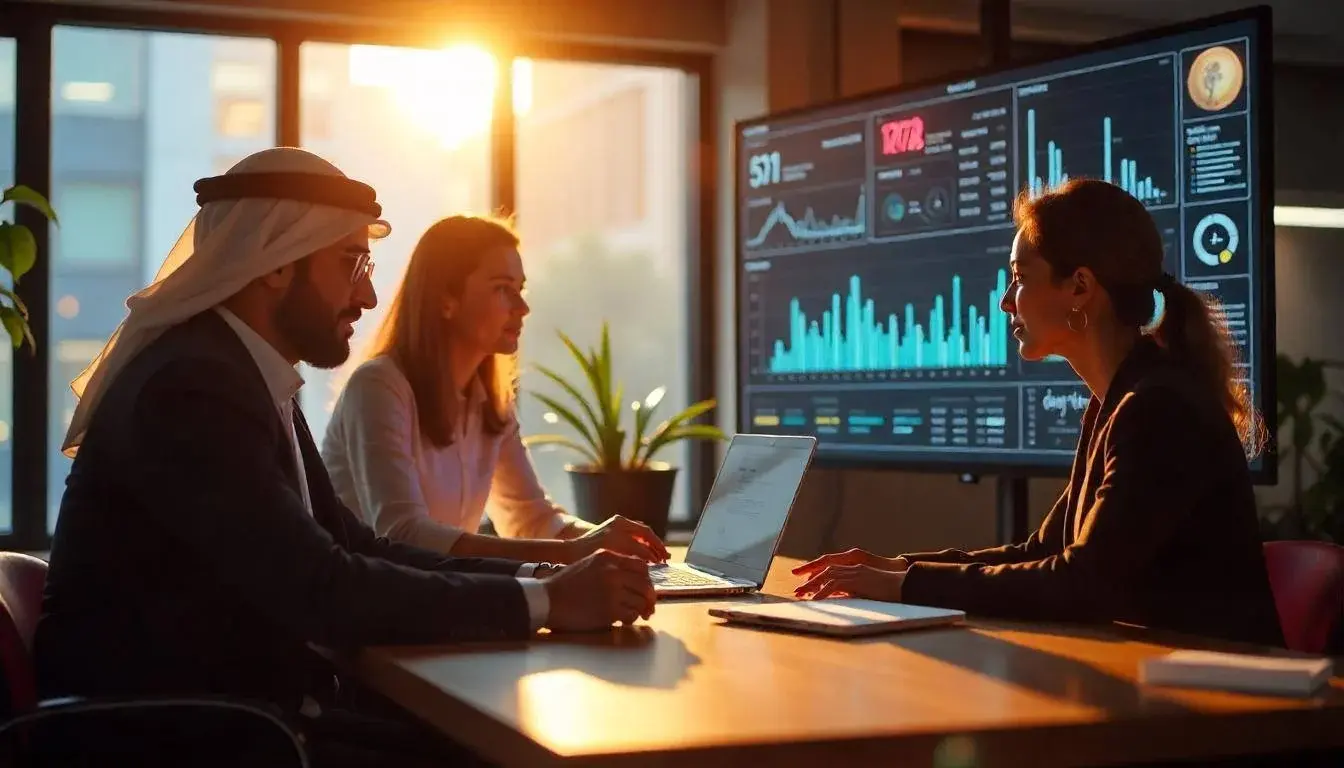
AI Means Business: How AI Can Create New Business Models
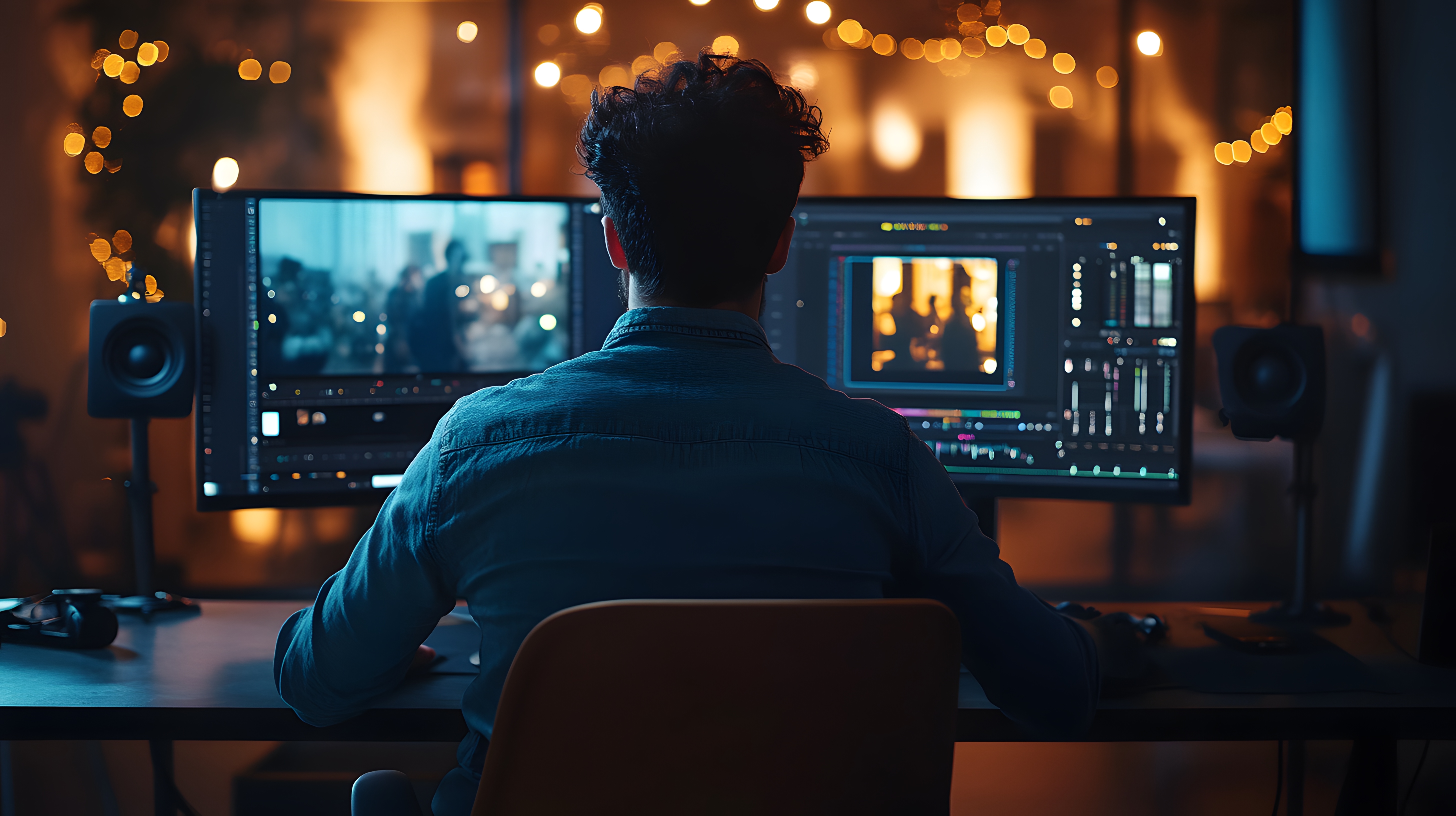
What Are Computer Vision Services? Definition and Use Cases
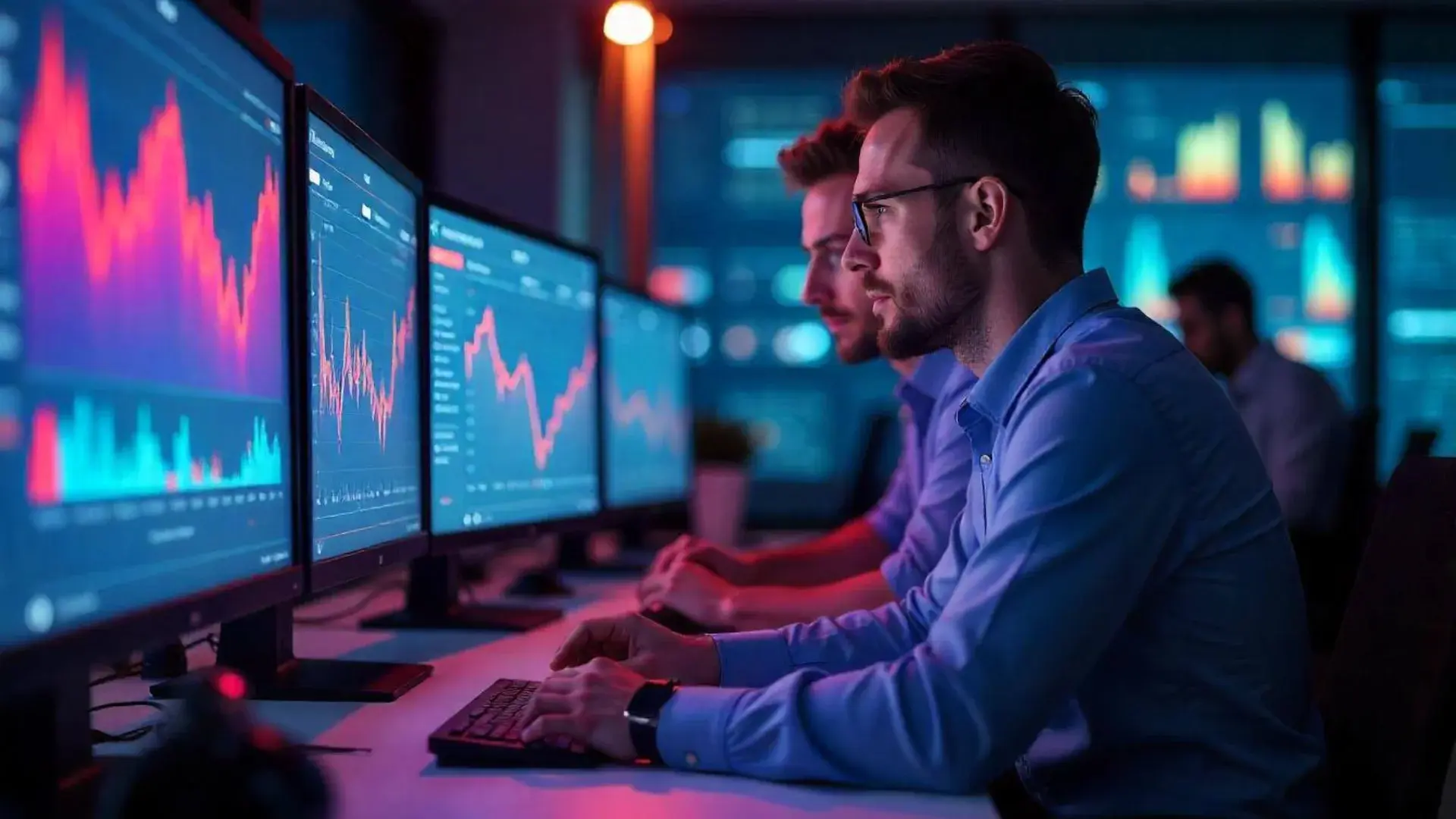
No Comments Yet
Let us know what you think